Simulated Annealing
Main.SimAnnealHW History
Hide minor edits - Show changes to markup
(:title Simulated Annealing Homework:)
(:title Simulated Annealing:)
(:description Homework on simulated annealing including a number of applications.:)
Simulated Annealing
Simulated annealing copies a phenomenon in nature--the annealing of solids--to optimize a complex system. Annealing refers to heating a solid and then cooling it slowly. Complete the following assignment using simulated annealing to arrive at an optimal solution.
(:description Exercise on simulated annealing for engineering optimization.:)
Simulated annealing is a probabilistic metaheuristic algorithm for approximating the global optimum of a given function. It is a search technique used in optimization for approximating the global optimum of a given function by mimicking the process of annealing in metallurgy. It works by randomly picking a solution from the search space and evaluating its cost, then randomly picking another solution and evaluating its cost and so on until an acceptable solution is found. The process is repeated multiple times with the aim of finding a global optimum solution.
Simulated annealing copies a phenomenon in nature (the annealing of solids) to optimize a complex system. Annealing refers to heating a solid and then cooling it slowly. Complete the following assignment using simulated annealing to arrive at an optimal solution.
(:html:)
<div id="disqus_thread"></div> <script type="text/javascript"> /* * * CONFIGURATION VARIABLES: EDIT BEFORE PASTING INTO YOUR WEBPAGE * * */ var disqus_shortname = 'apmonitor'; // required: replace example with your forum shortname /* * * DON'T EDIT BELOW THIS LINE * * */ (function() { var dsq = document.createElement('script'); dsq.type = 'text/javascript'; dsq.async = true; dsq.src = 'https://' + disqus_shortname + '.disqus.com/embed.js'; (document.getElementsByTagName('head')[0] || document.getElementsByTagName('body')[0]).appendChild(dsq); })(); </script> <noscript>Please enable JavaScript to view the <a href="https://disqus.com/?ref_noscript">comments powered by Disqus.</a></noscript> <a href="https://disqus.com" class="dsq-brlink">comments powered by <span class="logo-disqus">Disqus</span></a>
(:htmlend:)
% % Initialize DeltaE_avg if a worse solution was found % % on the first iteration
% Initialize DeltaE_avg if a worse solution was found % on the first iteration
% accept
% accept
(:toggle hide samatlab button show="Show Python Source":)
(:toggle hide samatlab button show="Show MATLAB Source":)
% disp(['Cycle: ',num2str(i),' trial: ',num2str(j)]);
% disp(['Cycle i:', num2str(i)]); % disp(['Trail j:', num2str(j)]);
% fprintf('xc(1) (in s. trial): %s \n',... % num2str(i),num2str(j),num2str(xc(1)));
% fprintf('xc(2) (in s. trial): %s \n',... % num2str(i),num2str(j),num2str(xc(2)));
% fprintf('fc (in s. trial): %s \n',... % num2str(i),num2str(j),num2str(fc));
% Store ACCEPTED candidates xc and corresponding solution f(xc)
% Record the best solution fa and corresponding candidates xa from the % stored values in the inner "trial" loop (at the end of every "trial"
(:toggle hide sapython button show="Show Python Source":) (:div id=sapython:) (:source lang=python:)
- Generate a contour plot
- Import some other libraries that we'll need
- matplotlib and numpy packages must also be installed
import matplotlib import numpy as np import matplotlib.pyplot as plt import random import math
- define objective function
def f(x):
x1 = x[0] x2 = x[1] obj = 0.2 + x1**2 + x2**2 - 0.1*math.cos(6.0*3.1415*x1) - 0.1*math.cos(6.0*3.1415*x2) return obj
- Start location
x_start = [0.8, -0.5]
- Design variables at mesh points
i1 = np.arange(-1.0, 1.0, 0.01) i2 = np.arange(-1.0, 1.0, 0.01) x1m, x2m = np.meshgrid(i1, i2) fm = np.zeros(x1m.shape) for i in range(x1m.shape[0]):
for j in range(x1m.shape[1]): fm[i][j] = 0.2 + x1m[i][j]**2 + x2m[i][j]**2 - 0.1*math.cos(6.0*3.1415*x1m[i][j]) - 0.1*math.cos(6.0*3.1415*x2m[i][j])
- Create a contour plot
plt.figure()
- Specify contour lines
- lines = range(2,52,2)
- Plot contours
CS = plt.contour(x1m, x2m, fm)#,lines)
- Label contours
plt.clabel(CS, inline=1, fontsize=10)
- Add some text to the plot
plt.title('Non-Convex Function') plt.xlabel('x1') plt.ylabel('x2')
- Simulated Annealing
- Number of cycles
n = 50
- Number of trials per cycle
m = 50
- Number of accepted solutions
na = 0.0
- Probability of accepting worse solution at the start
p1 = 0.7
- Probability of accepting worse solution at the end
p50 = 0.001
- Initial temperature
t1 = -1.0/math.log(p1)
- Final temperature
t50 = -1.0/math.log(p50)
- Fractional reduction every cycle
frac = (t50/t1)**(1.0/(n-1.0))
- Initialize x
x = np.zeros((n+1,2)) x[0] = x_start xi = np.zeros(2) xi = x_start na = na + 1.0
- Current best results so far
xc = np.zeros(2) xc = x[0] fc = f(xi) fs = np.zeros(n+1) fs[0] = fc
- Current temperature
t = t1
- DeltaE Average
DeltaE_avg = 0.0 for i in range(n):
print('Cycle: ' + str(i) + ' with Temperature: ' + str(t)) for j in range(m): # Generate new trial points xi[0] = xc[0] + random.random() - 0.5 xi[1] = xc[1] + random.random() - 0.5 # Clip to upper and lower bounds xi[0] = max(min(xi[0],1.0),-1.0) xi[1] = max(min(xi[1],1.0),-1.0) DeltaE = abs(f(xi)-fc) if (f(xi)>fc): # Initialize DeltaE_avg if a worse solution was found # on the first iteration if (i==0 and j==0): DeltaE_avg = DeltaE # objective function is worse # generate probability of acceptance p = math.exp(-DeltaE/(DeltaE_avg * t)) # determine whether to accept worse point if (random.random()<p): # accept the worse solution accept = True else: # don't accept the worse solution accept = False else: # objective function is lower, automatically accept accept = True if (accept==True): # update currently accepted solution xc[0] = xi[0] xc[1] = xi[1] fc = f(xc) # increment number of accepted solutions na = na + 1.0 # update DeltaE_avg DeltaE_avg = (DeltaE_avg * (na-1.0) + DeltaE) / na # Record the best x values at the end of every cycle x[i+1][0] = xc[0] x[i+1][1] = xc[1] fs[i+1] = fc # Lower the temperature for next cycle t = frac * t
- print solution
print('Best solution: ' + str(xc)) print('Best objective: ' + str(fc))
plt.plot(x[:,0],x[:,1],'y-o') plt.savefig('contour.png')
fig = plt.figure() ax1 = fig.add_subplot(211) ax1.plot(fs,'r.-') ax1.legend(['Objective']) ax2 = fig.add_subplot(212) ax2.plot(x[:,0],'b.-') ax2.plot(x[:,1],'g--') ax2.legend(['x1','x2'])
- Save the figure as a PNG
plt.savefig('iterations.png')
plt.show() (:sourceend:) (:divend:)
(:toggle hide samatlab button show="Show Python Source":) (:div id=samatlab:) (:source lang=matlab:) clc; clear; close all;
Generate a contour plot % Start location x_start = [0.8, -0.5];
% Design variables at mesh points i1 = -1.0:0.01:1.0; i2 = -1.0:0.01:1.0; [x1m, x2m] = meshgrid(i1, i2); fm = 0.2 + x1m.^2 + x2m.^2 - 0.1*cos(6.0*pi*x1m) - 0.1*cos(6.0*pi*x2m);
% Contour Plot fig = figure(1); [C,h] = contour(x1m,x2m,fm); clabel(C,h,'Labelspacing',250); title('Simulated Annealing'); xlabel('x1'); ylabel('x2'); hold on;
Simulated Annealing
% Number of cycles n = 50; % Number of trials per cycle m = 50; % Number of accepted solutions na = 0.0; % Probability of accepting worse solution at the start p1 = 0.7; % Probability of accepting worse solution at the end p50 = 0.001; % Initial temperature t1 = -1.0/log(p1); % Final temperature t50 = -1.0/log(p50); % Fractional reduction every cycle frac = (t50/t1)^(1.0/(n-1.0)); % Initialize x x = zeros(n+1,2); x(1,:) = x_start; xi = x_start; na = na + 1.0; % Current best results so far % xc = x(1,:); fc = f(xi); fs = zeros(n+1,1); fs(1,:) = fc; % Current temperature t = t1; % DeltaE Average DeltaE_avg = 0.0; for i=1:n
disp(['Cycle: ',num2str(i),' with Temperature: ',num2str(t)]) xc(1) = x(i,1); xc(2) = x(i,2); for j=1:m % Generate new trial points xi(1) = xc(1) + rand() - 0.5; xi(2) = xc(2) + rand() - 0.5; % Clip to upper and lower bounds xi(1) = max(min(xi(1),1.0),-1.0); xi(2) = max(min(xi(2),1.0),-1.0); DeltaE = abs(f(xi)-fc); if (f(xi)>fc) % % Initialize DeltaE_avg if a worse solution was found % % on the first iteration if (i==1 && j==1) DeltaE_avg = DeltaE; end % objective function is worse % generate probability of acceptance p = exp(-DeltaE/(DeltaE_avg * t)); % % determine whether to accept worse point if (rand()<p) % accept the worse solution accept = true; else % don't accept the worse solution accept = false; end else % objective function is lower, automatically accept accept = true; end
% disp(['Cycle: ',num2str(i),' trial: ',num2str(j)]);
% accept
if (accept==true) % update currently accepted solution
% disp(['Cycle i:', num2str(i)]); % disp(['Trail j:', num2str(j)]);
xc(1) = xi(1);
% fprintf('xc(1) (in s. trial): %s \n',... % num2str(i),num2str(j),num2str(xc(1)));
xc(2) = xi(2);
% fprintf('xc(2) (in s. trial): %s \n',... % num2str(i),num2str(j),num2str(xc(2)));
fc = f(xc);
% fprintf('fc (in s. trial): %s \n',... % num2str(i),num2str(j),num2str(fc));
% Store ACCEPTED candidates xc and corresponding solution f(xc) xa(j,1) = xc(1); xa(j,2) = xc(2); fa(j) = f(xc); % increment number of accepted solutions na = na + 1.0; % update DeltaE_avg DeltaE_avg = (DeltaE_avg * (na-1.0) + DeltaE) / na; else fa(j) = 0.0; end end % Record the best solution fa and corresponding candidates xa from the % stored values in the inner "trial" loop (at the end of every "trial" % cycle) fa_Min_Index = find(nonzeros(fa) == min(nonzeros(fa))); if isempty(fa_Min_Index) == 0 x(i+1,1) = xa(fa_Min_Index,1); x(i+1,2) = xa(fa_Min_Index,2); fs(i+1) = fa(fa_Min_Index); else x(i+1,1) = x(i,1); x(i+1,2) = x(i,2); fs(i+1) = fs(i); end % Lower the temperature for next cycle t = frac * t; fa = 0.0;
end % print solution disp(['Best candidate: ',num2str(xc)]) disp(['Best solution: ',num2str(fc)]) plot(x(:,1),x(:,2),'r-o') saveas(fig,'contour','png')
fig = figure(2); subplot(2,1,1) plot(fs,'r.-') legend('Objective') subplot(2,1,2) hold on plot(x(:,1),'b.-') plot(x(:,2),'g.-') legend('x_1','x_2')
% Save the figure as a PNG saveas(fig,'iterations','png') (:sourceend:)
Save as f.m
(:source lang=matlab:) function obj = f(x) x1 = x(1); x2 = x(2); obj = 0.2 + x1^2 + x2^2 - 0.1*cos(6.0*pi*x1) - 0.1*cos(6.0*pi*x2); end (:sourceend:) (:divend:)

Simulated annealing copies a phenomenon in nature--the annealing of solids--to optimize a complex system. Annealing refers to heating a solid and then cooling it slowly so that thermal equilibrium is maintained. Complete the following assignment using simulated annealing to arrive at an optimal solution.
Simulated annealing copies a phenomenon in nature--the annealing of solids--to optimize a complex system. Annealing refers to heating a solid and then cooling it slowly. Complete the following assignment using simulated annealing to arrive at an optimal solution.
Simulated annealing copies a phenomenon in nature--the annealing of solids--to optimize a complex system. Annealing refers to heating a solid to a liquid state and then cooling it slowly so that thermal equilibrium is maintained. Complete the following assignment using simulated annealing to arrive at an optimal solution.
Simulated annealing copies a phenomenon in nature--the annealing of solids--to optimize a complex system. Annealing refers to heating a solid and then cooling it slowly so that thermal equilibrium is maintained. Complete the following assignment using simulated annealing to arrive at an optimal solution.
(:title Simulated Annealing Homework:) (:keywords simulated annealing, Python, MATLAB:) (:description Homework on simulated annealing including a number of applications.:)
Simulated Annealing
Simulated annealing copies a phenomenon in nature--the annealing of solids--to optimize a complex system. Annealing refers to heating a solid to a liquid state and then cooling it slowly so that thermal equilibrium is maintained. Complete the following assignment using simulated annealing to arrive at an optimal solution.
Download these files to retrieve the latest versions of the example simulated annealing files in MATLAB and Python. These programs can serve as starting points for designing the homework solutions.
Download Simulated Annealing Example Files
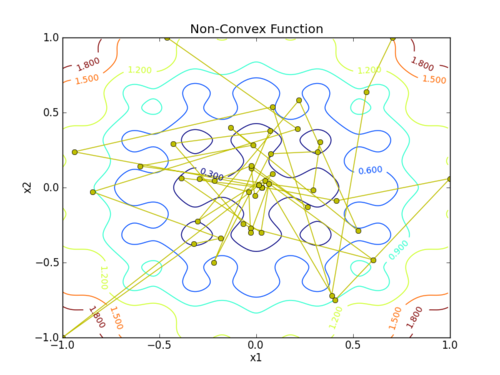
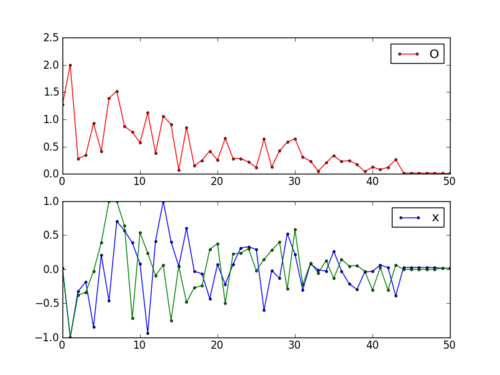
(:html:)
<div id="disqus_thread"></div> <script type="text/javascript"> /* * * CONFIGURATION VARIABLES: EDIT BEFORE PASTING INTO YOUR WEBPAGE * * */ var disqus_shortname = 'apmonitor'; // required: replace example with your forum shortname /* * * DON'T EDIT BELOW THIS LINE * * */ (function() { var dsq = document.createElement('script'); dsq.type = 'text/javascript'; dsq.async = true; dsq.src = 'https://' + disqus_shortname + '.disqus.com/embed.js'; (document.getElementsByTagName('head')[0] || document.getElementsByTagName('body')[0]).appendChild(dsq); })(); </script> <noscript>Please enable JavaScript to view the <a href="https://disqus.com/?ref_noscript">comments powered by Disqus.</a></noscript> <a href="https://disqus.com" class="dsq-brlink">comments powered by <span class="logo-disqus">Disqus</span></a>
(:htmlend:)